AQM Alumni

Tahsin Deniz Akturk
Tahsin Deniz Akturk started his doctoral study in the Booth School of Business in 2017 under the supervisions of Profs. Varun Gupta and Ozan Candogan. He is interested in asymptotic analysis, dynamic programming, inventory control, and mean-field models. As a student in the Operations area in Booth, Deniz has taken a variety of quantitative courses that focus on the mathematical theory and the applications in operations. He deems it important to see the applications of these tools in other contexts, and especially in various Social Sciences disciplines, and believes research that uses theoretical and empirical tools in such areas will expand his perspective as a researcher and help his academic career.
Through the certificate program, Deniz has gained a better understanding of the methodologies used across different areas and how these methodologies relate together, which would be very useful for his own research as it not only allows him to use some of these methods but also directs his research to important interdisciplinary questions. All 5 classes Deniz took for fulfilling the AQM requirements have been well taught and helpful in understanding the methodological foundations of research conducted across different disciplines. Among them, the stochastic process courses are especially valuable to him as they have provided a methodological basis for his own research. Deniz’s main advice for future AQM students is to try to participate AQM bi-weekly workshops presented by researchers from different departmental backgrounds as much as possible, as to him, “the best part about this program is that students have a chance to interact and learn from other disciplines, and future students should pursue this opportunity as much as possible.”
Deena Bernett
Deena Bernett is a doctoral student in Psychology who started her PhD study in 2015 with Prof. Susan Levine. Her interests include multilevel modeling and Bayesian statistics. While pursuing the AQM certificate program, Deena took several additional elective courses in advanced quantitative methods to better understand how to analyze her data or out of personal interest.
Minkwang Jang
Mingwang Jang is a doctoral student in the Booth School of Business and started his PhD study in 2018 to study with Profs. Oleg Urminsky and Ayelet Fishbach. His interests are Judgment and decision making, and decision modeling. Mingwang wanted to expand his quantitative skills beyond what is required or expected by his current program of study.

Jin Li
Jin Li is a BSD student who joined her PhD program in 2017 to study with Prof. Yun Fang. To Jin, the quantitative and methodological training that she received from the AQM program not only guided her to better design and execute her PhD research projects, but also provided her with new insight into this big data era. Jin believes that the Certificate will be a great demonstration of her quantitative skills that she built through her PhD studies and be an important complement to her degree in Biomedical Sciences.
Jin’s favorite elective course from AQM is MSCA 31009 Machine Learning and Predictive Analytics where she took it in summer evenings at the downtown NBC tower with Dr. Arnab Bose. The course is a well-designed introductory level machine learning course that explicitly covers fundamental concepts and methodologies on machine learning and deep learning, with abundant practical exercises to solidify our analytic skills. Jin believes UChicago is a great place to gain both fundamental and cutting-edge quantitative methods training as the university truly encourages PhD students from all fields to utilize these quantitative training resources, such as those offered by AQM, to expand knowledge and thinking boundaries.
Jin’s resarch interests include epigenetics, bioinformatics, metabolism, and cardiovascular diseases. One of her dissertation projects is to investigate how different types of hemodynamic flows remodel the epigenome and genome landscapes in endothelial cells contributing to atherosclerosis formation. After graduation, she would like to integrate her quantitative skills with her experimental specialization to conduct efficient and rigorous research in the biomedical fields.

Mengyuan Liang
Mengyuan Liang is a Ph.D. student with the Department of Comparative Human Development, studying under the supervision of Prof. Guanglei Hong. She decided to pursue the AQM certificate program because she has “always wanted to take advantage of the methodological courses across departments of UChicago, and have connections with other students and scholars who shared (similar) interests in statistical methods, which the program features.” She also believes that an AQM Certificate that she earns can be a solid proof of efforts in learning quantitative methods in graduate school. Indeed, the certificate program has not only encouraged Mengyuan to lay a solid foundation on statistical theories and hone her skills on applying the quantitative methods for various problems, but also enabled her to be a part of the research community that shares similar interests in advanced quantitative methods. Such experience and network will help in her future academic pursuits.
Reflecting on the coursework for the AQM certificate, Mengyuan considers Introduction to Causal Inference and STAT 245 to be her favorites. She would suggest future AQM students to “not be afraid of STAT 245; It might be painful but proved to be super helpful.”
Mengyuan’s dissertation is on the mediation role of teacher’s classroom instruction in the relationship between teacher’s content knowledge and student outcomes, and her current plan after graduation is to apply for jobs where she can apply her knowledge and skills to research and practice.

Simon Shachter
Simon Shachter jointed the Ph.D. program in Sociology in 2017 to work with Prof. Elisabeth S Clemens. His interests are nonprofits, philanthropy, political sociology, and organizations. Simon is specifically interested in the AQM program because there is a tremendous amount of data on nonprofits and philanthropy in the US, but there is still yet a way to turn that data into meaningful insights that can improve people’s lives. As a computer science major in his undergraduate study, Simon has a unique skill set to bring to these data questions to answer how the society helps people and how one can improve that system. To him, the AQM certificate is useful because it equips him with a suite of practical skills to engage in constructive discussions in developing quantitative evidence, and provides a foundation for him to share these skills to future students and collaborators. Through the courses, workshops, and conversations with faculty, he has developed a well-rounded knowledge and a way of approaching critical sociological questions.
Regarding the AQM coursework, Simon’s favorite was developing an understanding of the assumptions and limitations of different quantitative techniques, as each test statistic, table, or graph is layered with assumptions, research strategy, and broader social contexts. To him, “a presented number rarely means exactly what we want it to mean but, in many ways, also has a deeper significance.” He enjoys uncovering what a quantitative method actually reveals and all the things it does not, and wants to share with future AQM students that “unfortunately, there is a lot of gatekeeping concerning what it means to be, and who can be, a sophisticated and quantitatively-minded social scientist; Let’s try to break that down!”
Simon’s dissertation studies immigrant organizations and political power in West Coast cities in the 19th century. He is curious about the relations between immigrants as politics, as mediated by organizations, especially in place-based political development and creation of durable political structures and civil society; and is studying how immigrant groups (for various races, religions, and ethnicities) built power and had lasting effects on today’s institutions.

Xia Zheng
Xia Zheng is a Sociology major studying under the supervision of Professor Geoffrey Wodtke. She joined the PhD program in 2018 and her interests include economic inequality, causal inference, human capital, and machine learning. Xia believes that the AQM program provides her with not only essential training for conducting quantitative research but also the ability to learn new quantitative methods. Her favorite elective course for the AQM program is Applied Econometrics II, as the methods learned in class together with practice exercises allowed her to better understand a wide range of studies.

Likun Cao
Likun Cao joined the PhD program in SSD in 2019 to study with Prof. James Evans. Her interests include computational sociology, organizational analysis, innovation, and sociology of knowledge. As a young researcher in computational social science, Likun often uses statistical analyses in her research, and has found herself benefiting from the AQM curriculum. Specifically, the courses have extended her knowledge of quantitative methods while helping her familiarize with their practical applications; the workshops have been inspiring, equipping her with “insights from the academic frontiers.”
Likun’s favorite elective course in AQM was SOCI 40133 computational content analysis, which teaches students to use natural language tools to work with text data and solve social science problems. She believes that the soaring computational power and available data provide increasing possibilities for solving scientific puzzles. To Likun, the course was challenging, but she and each of her classmates have improved extensively in both coding skills and theoretical thinking.
To future AQM students, Likun says “just go for it!” She has sometimes felt exhausted due to workload from courses, but found it to be worthwhile when she applied the methodological tools to her project or came up with new ideas.

Liang Cai
Liang Cai
Liang Cai is a PhD candidate in Sociology at the University of Chicago. His research interest centers on how human behaviors are shaped by social and physical contexts and how causally relevant contexts should be defined. His recent work has explored activity space exposures, income segregation around the clock, and spatio-temporal patterns of crime. His ongoing projects examine 1. the spatial decision-making process and its social context in activity space, 2. how mobility patterns factor into individuals’ rural/urban living experiences, and 3. how social scientists can leverage computer vision AI to decipher local social ecology from street view images.
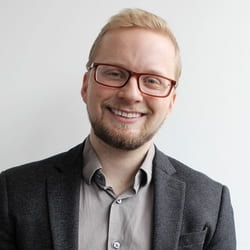
Jacy Anthis
Jacy Anthis
Jacy Anthis is a doctoral student pursuing a degree in Sociology as well as in Econometrics & Statistics. During his study with the AMQ program, he was able to use causal inference, machine learning, and other strategies to add richness and depth to social theories across almost every field. For Jacy, the AQM curriculum has helped him develop a more rigorous understanding of the social and moral dimensions of emerging technologies, especially with the 21st century Mississippi of digital exhaust data such as website texts amenable to natural language processing.
In his dissertation, Jacy studies the technical development and strategic management of artificial intelligence (AI). He has been working on how the sociocultural frames of AI have coevolved over time, technical research on how humans and algorithms can work together to make fair decisions, as well as experimental research on how humans assess different types of AI as collaborators and social actors. Jacy believes that “research on AI safety is increasingly important as these systems become more powerful and more pervasive in our society.”
Jacy’s favorite elective course in AQM is Victor Veitch’s seminar on Causal Inference with Machine Learning. As he states: “One of the most interesting aspects was how models of cause-and-effect could lead AI systems to be safer. Right now, leading paradigms in AI deep learning are very opaque, and by imposing some causal structures on the model, we might be able to better instruct them to avoid harm.” To Jacy, many of the most important questions in AI involve different methods for structuring advanced AIs built on deep learning.

Arvind Ilamaran
Ilamaran
Arvind Ilamaran is a Ph.D. student with the Department of Comparative Human Development. He holds a Master of Public Policy from the Harris School of Public Policy with a focus on education policy and causal inference methods. His dissertation research examines noncompliance in randomized experiments within a stochastic framework. His other works in progress are studying the impact of parental engagement on post-secondary completion and measuring the impact of childhood polyvictimization on adolescent academic and mental health outcomes. He has completed the Certificate of Sustainable Urban Development from the Mansueto Institute of Urban Innovation and held their doctoral fellowship during 2020-2021.

Isobel Heck
Heck
Isobel Heck started her PhD study in Psychology in 2016 at the Cornell University and transferred to the University of Chicago in 2019 with Prof. Katherine Kinzler. Her interests are on developmental psychology, social psychology, social status, and social hierarchies. Through taking statistics courses as a PhD student at Cornell, she have become increasingly aware of the benefits that a rich statistical toolkit can provide for tackling novel research questions. In her research, Isobel works with both children and adults, with a wide array of datasets (e.g., categorical, cross-sectional, longitudinal, nested, experimental, correlational). She hopes to use the AQM Certificate program to continue building her statistical toolkit, and gain deeper insight into the underlying theory supporting the statistical methods on a daily basis. Isobel also wants to connect with professors outside of her department who are experts in a variety of statistical methods.